News Story
Using underwater robots to detect and count oysters
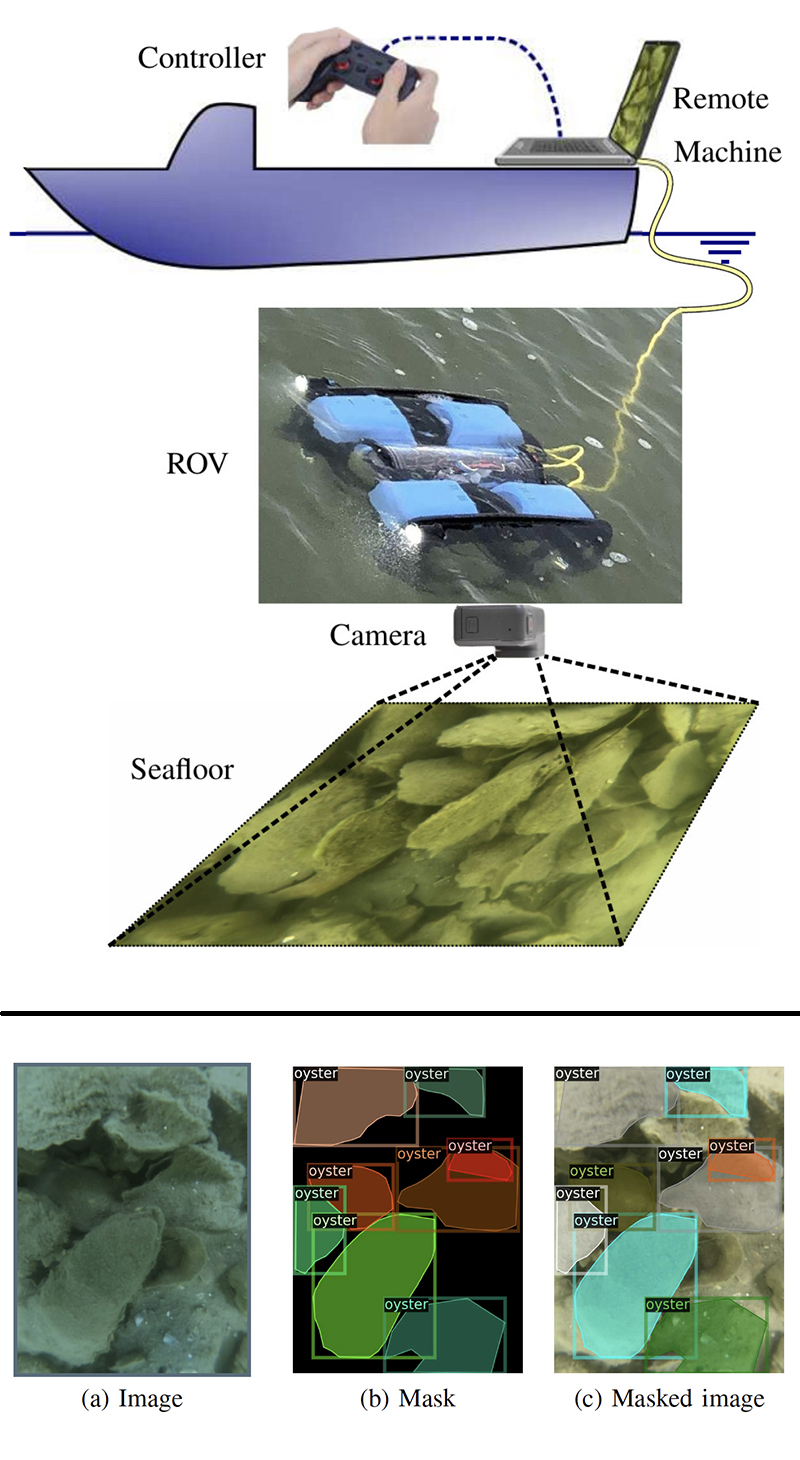
Top: Fig. 3 from the paper. A schematic image of how the robotic oyster counting system works. Bottom: Fig. 6 from the paper. A sample annotation and labeling of oysters in an image from the video taken by the robot vehicle.
Oysters, filter feeders that clean the surrounding water and create habitat for other species, are essential to the Chesapeake Bay and similar ecosystems. In the last century, oysters habitats have undergone various ecological stresses and have shrunken in size around the world.
While there are many ongoing projects to restore oysters habitats, it is challenging to determine how successful these efforts are. Measuring the numbers of oysters and their physical dimensions has always been performed manually and, is restricted to small numbers—for example, 100 oysters per sample site. These manual measurements are not regularly available for small-size aqua/oyster farms.
Now, advancements in robotics and artificial intelligence offer the potential to improve the monitoring process. Highly maneuverable, drone-like, remotely operated underwater vehicles (ROVs) are becoming affordable and can be used for sampling when fitted with proper sensory devices. Computer vision algorithms are being created to detect oysters and calculate their physical dimensions and properties. One of the most promising technologies is convolutional neural networks (CNNs), which can count oysters and track them in consecutive image frames so they are not identified multiple times.
New work by Maryland Robotics Center Postdoctoral Associate Behzad Sadrfaridpour (UMIACS), Professor Yiannis Aloimonos (CS/UMIACS), Professor Miao Yu (ME/ISR), Professor Yang Tao (BIOE), and Donald Webster, Principal Agent, UMD Wye Research and Education Center, is advancing this idea.
In their study, Detecting and Counting Oysters, the researchers customized a drone-like ROV, the BlueRobotics BlueROV2, with a GoPro HERO7 Black camera, for videography of the Chesapeake Bay bottom. They then worked with Bobby Leonard, a waterman, who took them out on his boat for tours of his oyster lease. The researchers used the ROV to produce videos of oysters in the Chesapeake Bay.
Afterwards, the team annotated the visual data captured from the bay bottom and created an oyster data set. Here they used a state-of-the-art CNN (Mask R-CNN) for target tracking and instance segmentation, and employed detectron2 for training and evaluating the oyster data set.
Their trained networks’ prediction results showed an Average Precision (AP50) of 81.8 for the oyster data set. They also combined the detection CNN with an object tracker for counting the number of oysters in videos.
The work will continue through 2024.
About the USDA NIFA shellfish project
This research is part of Transforming shellfish farming with smart technology and management practices for sustainable production, a five-year, $10M USDA National Institute of Food and Agriculture project headed by Miao Yu that includes researchers from the University of Maryland (UMD), the UMD Center for Environmental Science, the UMD Extension Service, the AGNR-UME-Sea Grant Extension, the University of Maryland Eastern Shore, Louisiana State University, the Pacific Shellfish Institute, and Virginia Tech.
The goal of this extensive project is to develop novel technologies and a sustainable management framework that can help farmers tap the economic potential and environmental benefits of shellfish aquaculture, perhaps the most ecologically sustainable form of aquaculture, as well as an important driver of coastal economy. The industry has been held back by its reliance on outdated tools and methods, but by synthesizing recent advances in robotics, agricultural automation, computer vision, sensing and imaging, and artificial intelligence, new ways can be developed to help enhance productivity and profitability, while better protecting fragile aquatic ecosystems.
| Read about the larger scope of the research project |
Published August 23, 2021