News Story
'OysterNet' + underwater robots will aid in accurate oyster count
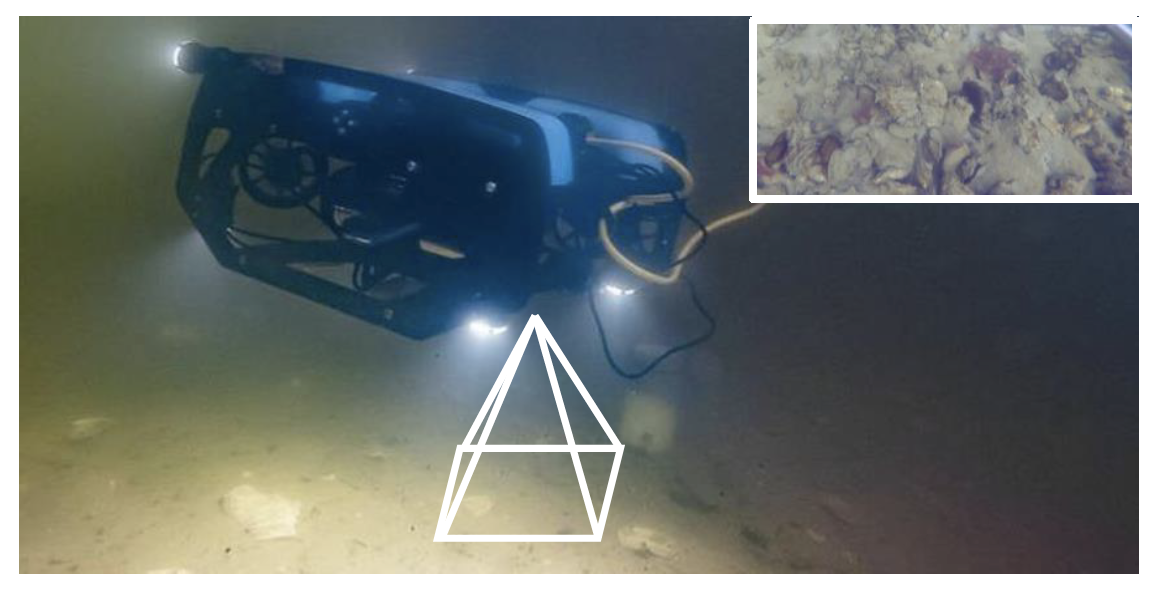
Here is the “BlueROV” robot collecting data for the real dataset. The inset shows a sample image captured, which is part of the real dataset. (Fig. 6 from the paper)
Oysters play a pivotal role in estuary ecosystems and are considered living filters for the ocean. Commercial over-harvesting, climate change and diseases have led to the devastation of oyster reefs, which now require preservation efforts to restore ecological balance.
The foundation of these preservation efforts is estimating oyster density, which requires accurate oyster detection and large datasets. Obtaining this information in underwater environments has always been an expensive and labor-intensive task.
In OysterNet: Enhanced Oyster Detection Using Simulation, Xiaomin Lin, Nitin J. Sanket, Nare Karapetyan, and ISR-affiliated Professor Yiannis Aloimonos (CS/UMIACS) present a new way to mathematically model oysters and render images of oysters in simulation. Their new method can boost detection performance with minimal real data, especially when used in conjunction with underwater robots.
The lead author, Xiaomin Lin, is an ECE Ph.D. student studying with Aloimonos. Nare Karapetyan is a UMD faculty assistant working in various robotics areas. Alum Nitin Sanket (CS Ph.D. 2021) is a former student of Aloimonos who recently completed a postdoctoral appointment at UMD and this fall began a tenure-track assistant professor position in robotics engineering at the Worcester Polytechnic Institute in Massachusetts.
The innovation
The researchers learned that using underlying geometrical properties of objects can help to enhance recognition task accuracy for limited datasets.
To streamline the process of oyster mapping, they took advantage of advancements in robotics and artificial intelligence. First, images taken by cameras on underwater robots—Remotely Operated Vehicles—were gathered. Then the researchers automated oyster detection and density calculation by building “OysterNet,” an oyster detection system that is the first attempt to model the 3D structure of oysters.
Fig. 3 from the paper (click image to enlarge): Steps in the geometric modelling of an oyster: (a) Sample image of a real oyster shell, (b) 3D scan of a real oyster, (c) Splines fit to the oyster’s bottom layer to model it (each color represents a single spline), (d) Simplified model of one stratified layer of the oyster (single layer of spline curve S(t)), (e) all layers of Sα(t), (f) generated 3D model of oyster, (g) final generated 3D model of oyster with added real oyster texture.
The researchers developed a mathematical model that could generate 3D oyster shapes, modeling the geometry of oyster shells and rendering the oyster images using a game engine. They simplified the geometric oyster model to project on an image plane, performed an image-to-image transformation from the simulation domain to the real-world domain, and generated photorealistic, synthetic oyster images.
These images then were used to train OysterNet. When the real dataset was augmented by the generated synthetic dataset, the team was able to obtain up to a 35.1% boost in performance compared to using only real data. They also improved the state of the art by 12.7%.
The results highlight that for data-critical applications where collecting real images is challenging or time-consuming, it is possible to model images using the underlying geometry of the object to create photorealistic images that drastically improve object detection.
About the USDA NIFA shellfish project
This research is part of Transforming shellfish farming with smart technology and management practices for sustainable production, a five-year, $10M USDA National Institute of Food and Agriculture project headed by Miao Yu that includes researchers from the University of Maryland (UMD), the UMD Center for Environmental Science, the UMD Extension Service, the AGNR-UME-Sea Grant Extension, the University of Maryland Eastern Shore, Louisiana State University, the Pacific Shellfish Institute, and Virginia Tech.
The goal of this extensive project is to develop novel technologies and a sustainable management framework that can help farmers tap the economic potential and environmental benefits of shellfish aquaculture, perhaps the most ecologically sustainable form of aquaculture, as well as an important driver of coastal economy. The industry has been held back by its reliance on outdated tools and methods, but by synthesizing recent advances in robotics, agricultural automation, computer vision, sensing and imaging, and artificial intelligence, new ways can be developed to help enhance productivity and profitability, while better protecting fragile aquatic ecosystems.
| Read about the larger scope of the research project |
Published October 21, 2022