News Story
Electric power grid signatures may aid in image and video verification
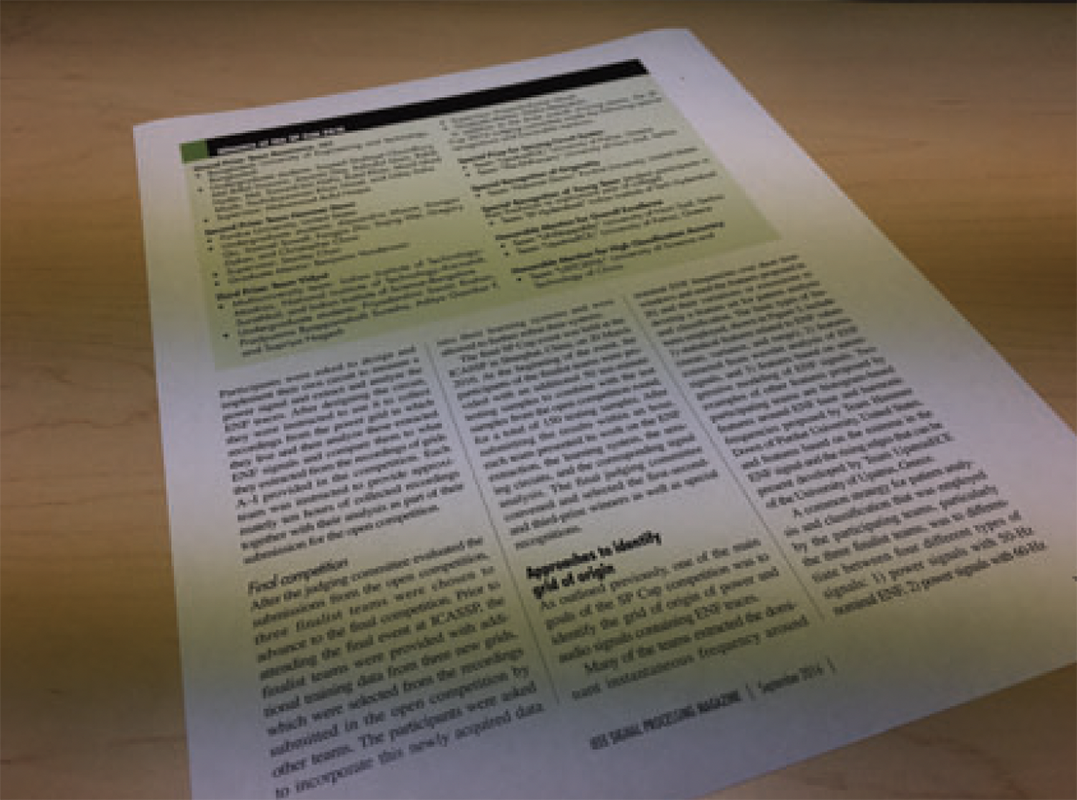
An ENF trace signature can easily be seen in this digital image of a piece of printed paper. Figure 1(a) from the current research, "Invisible Geolocation Signature Extraction from a Single Image."
As video and still images about Ukraine flood cable television, Internet news sources and social media channels, verifying their authenticity is of utmost importance. Before using them, responsible news organizations have been spending considerable time and resources on identifying dates, locations, and what actually is taking place in the images. But bad actors are still able to get many old, faked, deliberately mislocated and misidentified graphics out to the world where viewers take them at face value.
Digital media contain many metadata fields, one of which can relate to location information using built-in GPS input. This piece of metadata, however, is not always available and can be easily altered. Many images do not carry trustworthy and tamper-resilient location tags. In addition, visual clues that are often relied upon such as terrain and landmarks may not be readily present in every image, especially in those taken indoors. So questions remain: Where and when was a hostage video made? Is an image of a protest genuine, or is it actually from a different place and time? Was sound added to a video after the fact?
ISR-affiliated Professor Min Wu (ECE/UMIACS) is a specialist in digital media forensics, including new ways to verify the authenticity of digital assets like videos and images. For more than a decade, she has been exploring how an almost-unnoticeable, location-related environmental signature from electric power grids inherently embedded in images and videos can capture useful time/location information. This signature, called the electric network frequency (ENF) signal, is part of a sensing stream at the time of recording.
Researchers recently have learned how to extract ENF traces from audio and video recordings. However, it is more challenging to extract an ENF trace from a single image, especially in scenarios that existing technologies cannot sufficiently address.
Along with her former student Chau-Wai Wong (ECE Ph.D. 2017), now an assistant professor at North Carolina State University and the lead for this work; his Ph.D. student Jisoo Choi; her former student Adi Hajj-Ahmad (ECE Ph.D. 2016), now a data scientist with Amazon Alexa; and Yanpin Ren, Tsinghua University, China; Wu has written a new paper that addresses this challenge, “Invisible Geolocation Signature Extraction from a Single Image.”
The ENF signal comes from the behavior of the power distribution network, which has a varying frequency that reflects the behavior of the power grid at the time and the location where the media recording was captured. This relationship enables the time, location, and integrity estimation of corresponding multimedia recordings. To produce one frequency estimate, audio/video recordings need a signal that is several seconds long. Wu and her colleagues wondered whether there was a way for ENF traces to be captured in a single image taken at one moment in time, whether it would be possible to detect them, and whether the traces could be narrowed to 50 vs. 60 Hz.
They addressed an image’s location by first mathematically examining the impact of ENF embedding steps such as electricity to light conversion, scene geometry dilution of radiation, and image sensing. They then incorporated verified parametric models of the physical embedding process into an entropy minimization method. The optimized results were used to create a two-level ENF presence–classification test that could identify whether a single image has an ENF trace. If the answer is “yes,” the test is able to show whether it is at 50 or 60 Hz. The authors also quantitatively studied the relationship between the ENF strength and its detectability from a single image.
The paper is the first comprehensive work to develop a unique forensic capability of environmental traces to geotag individual images that do not have a visible landmark or GPS tag, especially those captured indoors. The new capability can help shed light on an image’s capturing location.
Beyond multimedia, ENF is present in other forms of sensings and lends itself naturally to addressing security needs in the fast-progressing area of Internet of Things (IoT), as Wu’s 2017 paper at a leading conference on IoT envisioned. Wu currently is working with ECE Assistant Professor Sahil Shah, a circuit and power system expert, to explore the challenges and opportunities in this domain.
Related work
Feasibility Study on Intra-Grid Location Estimation Using Power ENF Signals (2021) by Min Wu’s former student Ravi Garg (ECE Ph.D. 2013), a Senior Applied Scientist at Amazon; Adi Hajj-Ahmad; and Min Wu.
New Directions: Proof-Carrying Sensing – Towards Real-World Authentication in Cyber-Physical Systems, by Min Wu, F.M. Quintão Pereira, J. Liu, H.S. Ramos, M.S. Alvim, and L.B. Oliveira, in the Proceedings of ACM Conf. on Embedded Networked Sensor Systems (SenSys), Delft, Netherlands, Nov. 2017.
Geo-Location Estimation from Electrical Network Frequency Signals (2013) by Ravi Garg, Adi Hajj-Ahmad, and Min Wu, presented at the 2013 IEEE International Conference on Acoustics, Speech and Signal Processing (ICASSP 13).
"'Seeing' ENF: Natural Time Stamp for Digital Video via Optical Sensing and Signal Processing," a paper by Ravi Garg, A.L. Varna and Min Wu, was published in IEEE Transactions on Information Forensics and Security in 2013. An earlier version of this paper won the Best Student Paper Award at ACM Multimedia 2011.
Published April 14, 2022