News Story
Entity resolution tool useful to uncover new information in 'big data' sets
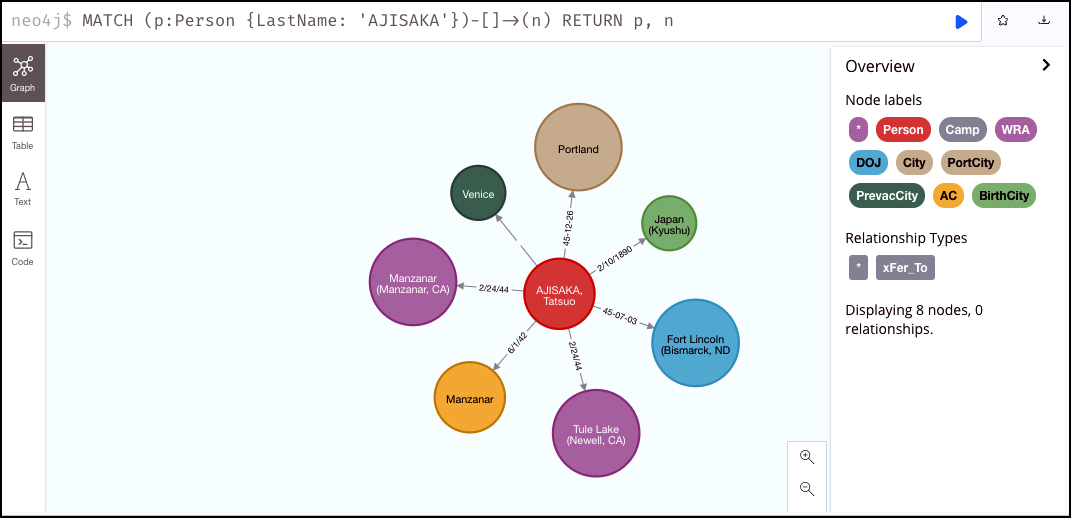
Fig. 8 from the paper illustrates how a query on "Tatsuo Ajisaka" reveals major life and incarceration events including: birthplace (light green node: Kyushu, Japan), pre-evacuation city (dark green: Venice, California), Temporary Assembly Center of entry (orange node: Manzanar), 1st WRA Camp (purple node: Manzanar), 2nd and final WRA Camp (purple node: Tule Lake), DOJ Camp of deportation (light blue node: Fort Lincoln, Bismarck ND), and finally Port City from which he was deported back to Japan (light brown node: Portland, OR).
“Big data” is a term used to describe large, complex data sets, especially from new data sources. These data sets are so large that traditional data processing software cannot manage them. Big data is defined by “the three ‘Vs’”: high volumes of low-density, unstructured data, high velocity (the rate at which more data is added to the set), and high variety (the many different types of data in the set). Information scientists are developing new ways to analyze, extract information from, and otherwise deal with these large, complex sets.
A new tool called Entity Resolution (ER) is increasingly being used to identify and link/group different manifestations of the same real-world object across archival collections. Examples where ER is being applied include linking Census records, counter terrorism, and spam detection. ER is also known as record linkage, reference reconciliation, and approximate match, among others.
In a paper presented at the 2021 IEEE International Conference on Big Data, the ER technique known as “fuzzy matching” and other computational approaches are used to unlock and link biographical data from WWII Japanese American incarceration camps.
A Framework for Unlocking and Linking WWII Japanese American Incarceration Biographical Data was written by ISR-affiliated Professor Richard Marciano, College of Information Studies (CIS); CIS Senior Faculty Specialist Greg Jansen; Lencia Beltran, UNC Wilmington; and Emily Ping O’Brien, Worcester Polytechnic Institute.
The project explores digital history methods based on Computational Archival Science (CAS) that use large quantities of data for historical research. In methodological terms, the authors focus on aspects of prosopography, a means of profiling any group of recorded persons linked by any common factor.
The authors demonstrate the construction of social graphs that are able to link people, places, and events; support further scholarship; and reveal hidden stories in historical events, especially in contested archival sources. They also show the power of computational analysis to recreate event networks and represent movements of people using maps.
The modeling is captured through interactive Jupyter Notebooks that integrate the various elements and document the interpretation of Japanese American experiences and events at the Tule Lake internment camp.
The work is made possible in part because of newly released archival sources of the WWII Japanese-American incarceration that previously had not been publicly available. The authors focus on more than 25,000 narrative reports prepared by camp investigators, police officers, and directors of internal security, relating cases of alleged "disorderly conduct, rioting, seditious behavior" at each of the 10 major internment camps. The reports include detailed information on the names and addresses in the camps of the persons involved, the time and place where the alleged incident occurred, an account of what happened, and a statement of action taken by the investigating officer, and even photos.
By processing these records by extracting their information content, and creating models of people and events across space and time, a potential "history-changer" picture emerges that could unlock a more complete story of what happened in the camps. In our current historical moment of heightened attention to misinformation, the project also highlights the transformation of information to knowledge in a way that can deepen understanding.
Published February 10, 2022