News Story
New reinforcement learning framework for biophamaceutical manufacturing
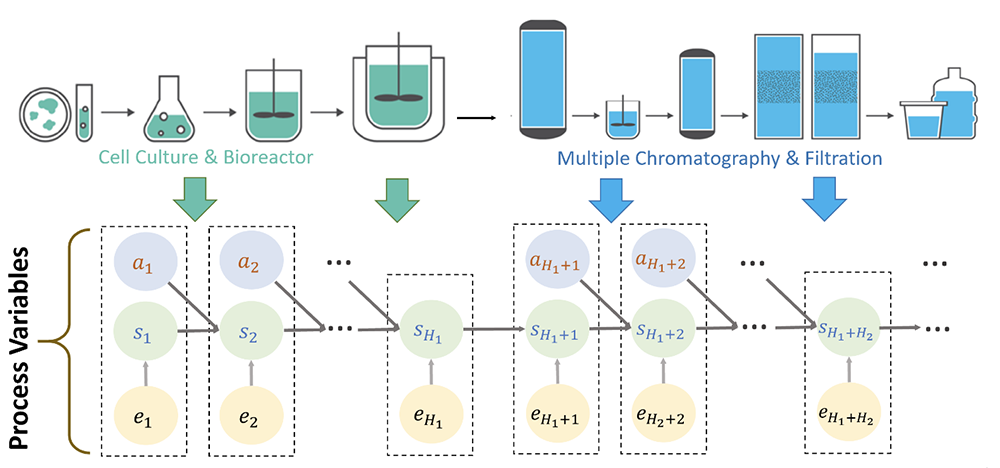
Illustrative example of network model for an integrated biopharmaceutical production process. (Fig. 1 from the paper)
Biopharmaceutical manufacturing is a rapidly growing industry (more than $300 billion in revenue in 2019) that affects virtually all branches of medicine. Biomanufacturing processes are complex and dynamic, involve many interdependent factors, and are performed with extremely limited data due to the high cost and long duration of experiments. Process control is critical to the success of the industry.
In new work, Associate Professor Ilya Ryzhov (BMGT/ISR) and his colleagues at Northeastern University and the University of Massachusetts Lowell, have developed a model-based reinforcement learning framework that greatly improves the ability to control bioprocesses in low-data environments.
Policy Optimization in Bayesian Network Hybrid Models of Biomanufacturing Processes was written by Hua Zheng and Wei Xie of Northeastern University, Ilya Ryzhov, and Dongming Xie of the University of Massachusetts Lowell and is available on the website arXiv.org.
The model uses a probabilistic knowledge graph to capture causal interdependencies between factors in the underlying stochastic decision process. It leverages information from existing kinetic models in different unit operations while incorporating real-world experimental data. The researchers also present a computationally efficient, provably convergent stochastic gradient method for policy optimization and conduct validation on a realistic application with a multi-dimensional, continuous state variable.
About pharmaceutical biomanufacturing
More than 40% of the products in the pharmaceutical industry’s development pipeline are bio-drugs, designed for prevention and treatment of diseases such as cancer, Alzheimer’s disease, and most recently COVID-19. These drugs are manufactured in living cells whose biological processes are complex and highly variable.
A typical biomanufacturing production process consists of numerous unit operations, e.g., cell culture, purification, and formulation, each of which directly impacts the outcomes of successive steps. To improve productivity and ensure drug quality, the process must be controlled as a whole, from end to end. In this industry, production processes are very complex, and experimental data are very limited, because analytical testing times for complex biopharmaceuticals are very long.
Unfortunately, “big data” do not exist; in a typical application, a process controller may have to make decisions based on 10 or fewer prior experiments. Not surprisingly, human error is frequent in biomanufacturing, accounting for 80% of deviations.
Innovations of the research
The new optimization framework is based on reinforcement learning (RL), which demonstrably improves process control in the small-data setting. The researchers’ approach incorporates domain knowledge of the underlying process mechanisms, as well as experimental data, to provide effective control policies that outperform both existing analytical methods as well as human domain experts.
Biomanufacturing has traditionally relied on deterministic kinetic models, based on ordinary or partial differential equations, to represent the dynamics of bioprocesses. Classic control approaches for such models, such as feed-forward, feedback, and proportional-integral-derivative control, tend to focus on the short-term impact of actions while ignoring their long-term effects. They also do not distinguish between different sources of uncertainty, and are vulnerable to model misspecification. Furthermore, while these physics-based models have been experimentally shown to be valuable in various types of biomanufacturing processes, an appropriate model may simply not be available when dealing with a new biodrug.
These limitations have led to recent interest in models based on Markov decision processes, as well as more sophisticated deep reinforcement learning approaches. However, these techniques fail to incorporate domain knowledge, and therefore require much greater volumes of training data before they can learn a useful control policy. Additionally, they have limited interpretability, and their ability to handle uncertainty is also limited. Finally, existing studies are limited to individual unit operations and do not consider the entire process from end to end.
The researchers’ new framework takes the best from both worlds. Unlike existing papers on RL for process control, which use general-purpose techniques such as neural networks, their approach is model-based. They create a knowledge graph that explicitly represents causal interactions within and between different unit operations occurring at different stages of the biomanufacturing process.
This hybrid model simultaneously incorporates real-world data as well as structural information from existing kinetic models. Using Bayesian statistics, the researchers quantify model uncertainty about the effects of these interactions. The approach is validated in a realistic case study using experimental data for fed-batch fermentation of Yarrowia lipolytica, a yeast with numerous biotechnological uses. As is typical in the biomanufacturing domain, only eight prior experiments were conducted, and these are the only data available for calibration and optimization of the production process. Nonetheless, by combining these data with information from existing kinetic models, the new DBN-RL framework achieves human-level process control with a fairly small number of training iterations, and outperforms the human experts when allowed to run longer. The approach is more data-efficient than a state-of-the-art model-free RL method.
This approach is especially effective in engineering problems with complex structure derived from physics models. Such structure can be partially extracted and turned into a prior for the Bayesian network. Mechanistic models can also be used to provide additional information, which becomes very valuable in the presence of highly complex nonlinear dynamics with very small amounts of available pre-existing data.
All of these issues arise in the domain of biomanufacturing. The researchers demonstrated that their approach can achieve human-level control using as few as eight lab experiments, while state-of-the-art model-free methods struggle due to their inability to incorporate known structure in the process dynamics.
As synthetic biology and industrial biotechnology continue to adopt more complex processes for the generation of new drug products, from monoclonal antibodies (mAbs) to cell/gene therapies, data-driven and model-based control will become increasingly important. Model-based reinforcement learning can provide competitive performance and interpretability in the control of these important systems.
Published May 20, 2021