News Story
NEXTOR III research helping general aviation airports track usage
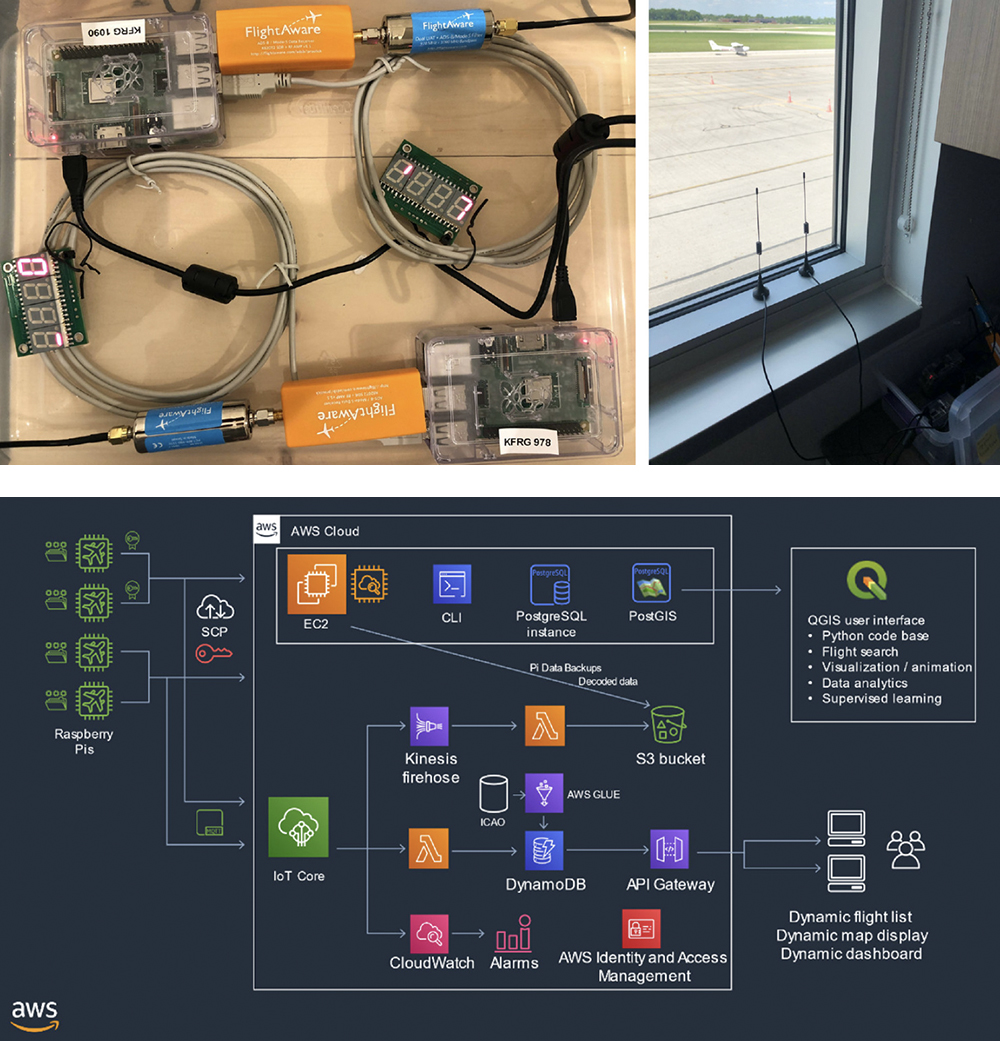
The United States is home to thousands of small “general aviation” (GA) airports that see unique, unpredictable and frequently changing amounts of activity. Weather conditions and wind direction play outsized roles; on some days runways are used heavily, on others hardly at all. Flight schools often operate out of general aviation airports, and their aircraft perform landings, takeoffs, and touch-and-goes multiple times per training session. Also, in general smaller aircraft make many trips per day and frequently change their flight patterns.
Unlike large commercial airports, GA airports usually do not have control towers directing and monitoring flights and automatically collecting aircraft operations data. This makes collecting and parsing operations information more difficult and complex. At the same time, gathering and interpreting such information is especially important for GA airports. They need reliable and accurate information on usage because they rely on federal funding to maintain and improve their capacity levels.
In Developing Capacity Estimation Metrics for Airports Accommodating Smaller Aircraft Using Locally Collected Automated Dependent Surveillance-Broadcast Data, researchers from the University of Maryland and Ohio State University used home-built automated dependent surveillance-broadcast (ADS-B) data collection units to develop aircraft performance characteristics that could be used to model small airport capacity. The paper was published in the June 2022 issue of Transportation Research Record, the journal of the Transportation Research Board of the National Academies.
The paper’s authors are Professor David Lovell (CEE/ISR); Lovell’s former student and alum Danae Zoe Mitkas (CEE M.S. 2021), now with the Federal Aviation Administration; and Seth Young and Sandeep Venkatesh of the Ohio State University.
All are associated with NEXTOR III, the eight-university FAA Consortium in Aviation Operations Research contracted by the Federal Aviation Administration to support research on a wide range of aviation topics. NEXTOR III generates new ideas and paradigms in air transportation by educating and training aviation professionals and promoting knowledge transfer among industry, government and academic leaders.
About the research
The researchers sought to leverage self-collected ADS-B data in order to extract location-related capacity metrics. They built ADS-B data collection units using a Raspberry Pi-based hardware platform and an Amazon Web Services-based cloud architecture to house the study’s PostGreSQL database, and python-based codebase. These units were deployed at three study airports: The Ohio State University Airport in Columbus, Ohio (KOSU); the College Park, Md., Airport (KCGS); and Republic Airport in Farmingdale, N.Y. (KFRG).
During the study period, the units collected more than 90 million individual ADS-B messages transmitted by aircraft operating within the vicinity of the airports. The researchers then developed models to clean, process, and assess the data, and leveraged them to determine aircraft performance characteristics.
The data enabled the researchers to assess fleet mix, approach speeds, aircraft separation in arrival streams, and runway occupancy times for each airport.
The units returned highly accurate and rich collected and processed data. The performance metrics were reasonable, valid, and applicable for use as inputs to future airport capacity models.
The method was found to be an extremely useful tool for smaller airports that face the need to count operations and monitor capacity levels, but do not have the necessary facilities to do so. Because the data were collected and analyzed autonomously, they exhibited a high degree of authenticity. The results indicate that locally collected automated dependent surveillance-broadcast (ADS-B) data indeed can estimate aircraft performance characteristics and contribute to enhanced airport capacity models for GA airports.
Future research
The research could be expanded by investigating performance characteristics based on specific runway operating environments, and by exploring specific meteorological conditions such as wind direction and speed, and cloud ceiling and visibility conditions. Especially for locations where inclement weather conditions are frequent and the option to shift operations from one runway to another to avoid strong crosswinds is not available, this additional analysis will provide significant insights. In addition, the researchers hope to produce counts per operation type for each airport. A machine learning model could be used to parse each flight into the number of operations.
With additional modeling efforts, the use of ADS-B data could be effective in creating the data inputs to models that will more accurately estimate the capacity of airports serving small GA aircraft, helping these airports secure the federal funding needed to sustain and improve their capacities.
Published September 1, 2022