News Story
AI and ML techniques can help unlock hard-to-reach archival content from pre-digital age
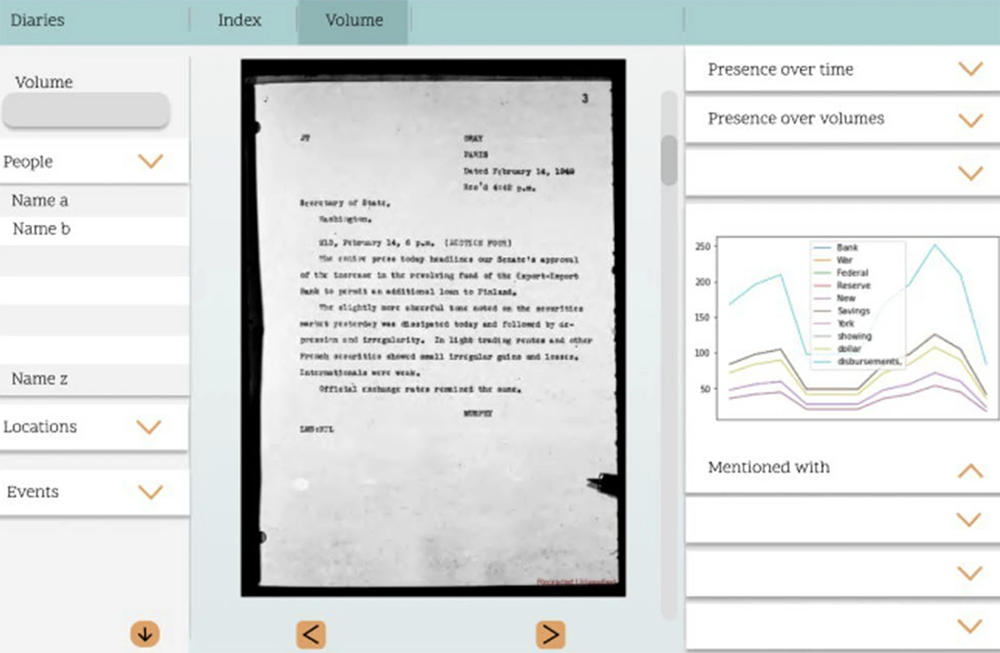
CLICK FOR LARGER VIEW. Fig. 15 from the paper. The user interface will index and display diary contents down to the document level.
ISR-affiliated Professor Richard Marciano (College of Information Studies) recently brought together archivists, scholars, and technologists to demonstrate computational treatments of digital cultural assets using artificial intelligence (AI) and machine learning (ML) techniques to help unlock hard-to-reach archival content.
Marciano worked with Kirsten Carter and Abby Gondek of the Roosevelt Institute and Franklin D. Roosevelt Presidential Library and Museum in Hyde Park, N.Y.; William Underwood, an Adjunct Research Scientist in the College of Information Studies; and Teddy Randby, a full stack software engineer in the Advanced Information Collaboratory (AIC).
The team engaged in an extended, iterative study applied to digitized and datafied WWII-era records housed at the FDR Presidential Library, rich content that is regrettably under-utilized by scholars examining American responses to the Holocaust. In particular, they worked with the extensive diaries of U.S. Treasury Secretary, Henry Morgenthau, Jr.—864 bound volumes that represent 12 years of daily business records. The diaries provide a focused, yet complex, textual corpus.
Their resulting paper, Using AI and ML to optimize information discovery in under-utilized, Holocaust-related records, illustrates the efforts, including trial and error, to extract and augment data drawn from the Morgenthau Diaries’ original custom indexing system first developed and used by the Secretary’s own office, combined with more recent digitized image data and descriptive structures imposed by archivists. The work has been published in the Springer open access journal AI & Society.
Digital cultural assets are often thought to exist in separate spheres based on their two principal points of origin: those that have been digitized and those created digitally. Increasingly, advances in digital curation are blurring this dichotomy, by introducing so-called “collections as data,” which regardless of their origination make cultural assets more amenable to the application of new computational tools and methodologies.
The authors identify and apply tools and methodologies (including ML through object detection and AI through “Named Entity Recognition”), reaching the real-world outcome of public access to augmented data. The study also discusses issues of digital representation, relational context, and interface design to enable new modes of public and scholarly access. The work is a substantial contribution to revealing the strengths and weaknesses of using AI/ML systems in cultural organizations.
Such datafication and augmentation efforts should culminate in improved access and usability outcomes for the public and data scholars, engendering deeper human understanding of collections, inspiring and sustaining new and more complex historical inquiries, and unlocking the potential for new modes of interpretive analysis.
About the Advanced Information Collaboratory
While the use AI and ML in historical archives is in its exploratory stage, it represents an emerging trend being tried by number of cultural organizations, including: Yad Vashem, the World Holocaust Remembrance Center, The Smithsonian Institution Data Science Lab in collaboration with the United States Holocaust Memorial Museum (USHMM), the European Holocaust Research Infrastructure (EHRI) project, the Library of Congress “Newspaper Navigator” Dataset, The National Archives (UK), and many others.
In Feb. 2020, Marciano, Underwood and colleagues launched the Advanced Information Collaboratory (AIC) with partners from leading academic and cultural institutions. AIC’s emphasis is to explore the opportunities and challenges of “disruptive technologies” for archives and records management, such as AI, ML and Computational Archival Science (CAS). These technologies will help to unlock the hidden information in massive stores of records. Recently, the AIC launched a targeted AI/ML/CAS initiative called the Future of Archives and Records Management (FARM).
This paper represents the first FARM Initiative case study to explore using ML strategies to apply predictive modeling in the extraction of valuable hidden archival materials.
Published August 29, 2022