News Story
Maryland researchers awarded DARPA cooperative agreement to develop robotic swarm strategies
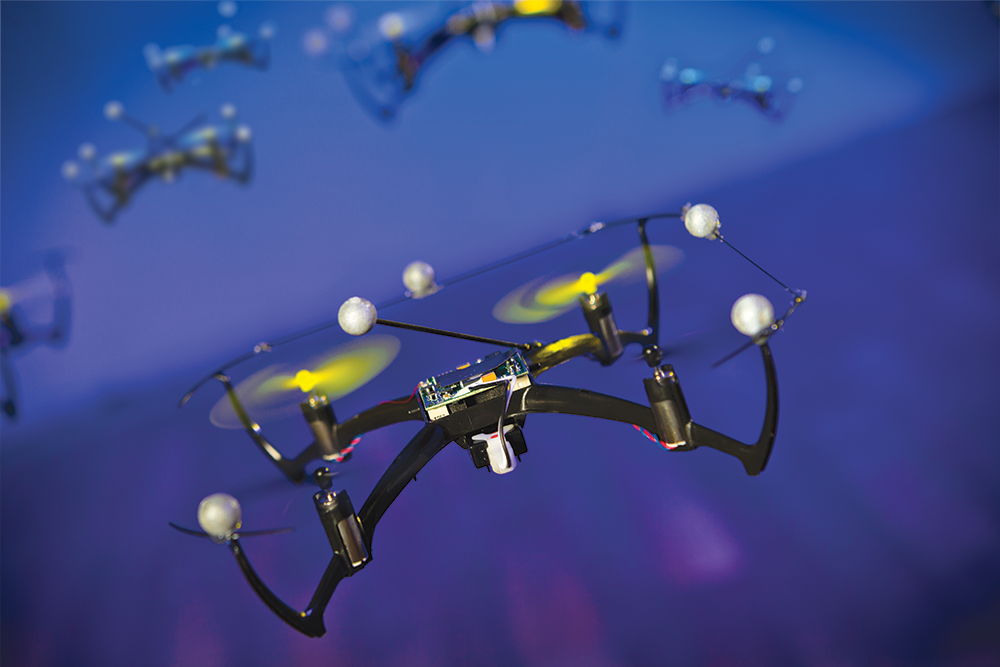
A team of four University of Maryland researchers has been awarded a nine-month, $646K cooperative agreement from the Defense Advanced Research Projects Agency (DARPA) for “Robust Semi-Autonomous Swarm Tactics for Situational Awareness in Uncertain Environments.” Assistant Professor Huan Xu (AE/ISR) is the principal investigator. The team also includes Assistant Professor Michael Otte (AE); Dinesh Manocha (CS/ECE/UMIACS), who will soon join the university as the Paul Chrisman Iribe Chair of Computer Science and Electrical and Computer Engineering; and Ming Lin (CS/UMIACS), the Elizabeth Stevinson Iribe Chair of Computer Science.
The grant is among the first “core swarm sprints” awarded in DARPA’s OFFensive Swarm-Enabled Tactics (OFFSET) program. In addition to the University of Maryland, the cohort includes Carnegie Mellon University, Lockheed Martin Advanced Technology Laboratories, SoarTech, Inc., and Charles River Analytics, Inc.
The OFFSET program is funding “swarm” autonomy and human-swarm teaming technology research to improve the capabilities of groups of aerial and ground robots that work together to help humans accomplish tasks such as surveillance in complex and changing urban settings.
In current drone surveillance situations, robot teams are limited in number, use “one-size-fits-all” surveillance strategies, and assume the environment is static. However, since environments are complex and change frequently, better surveillance strategies are needed.
The Maryland researchers will combine multiple existing autonomous surveillance strategies into a single framework, enabling simultaneous overlapping deployment in different subsets of the environment. They will produce a set of semi-autonomous aerial swarm tactics that can be used simultaneously in different parts of the environment and will account for uncertainties, sensor errors and dynamic obstacles. The goal is to develop and evaluate new swarm simulation algorithms that can automatically adapt to the complexity of urban environments.
Humans that use such a system would be able to fine-tune three different semi-autonomous surveillance strategies, which would lead to improved surveillance capabilities in urban environments.
The researchers will develop their strategies during the first six months of the project. In the final three months these strategies will be tested on robotic platforms.
Published April 3, 2018