News Story
A learning algorithm for training robots' deep neural networks to grasp novel objects
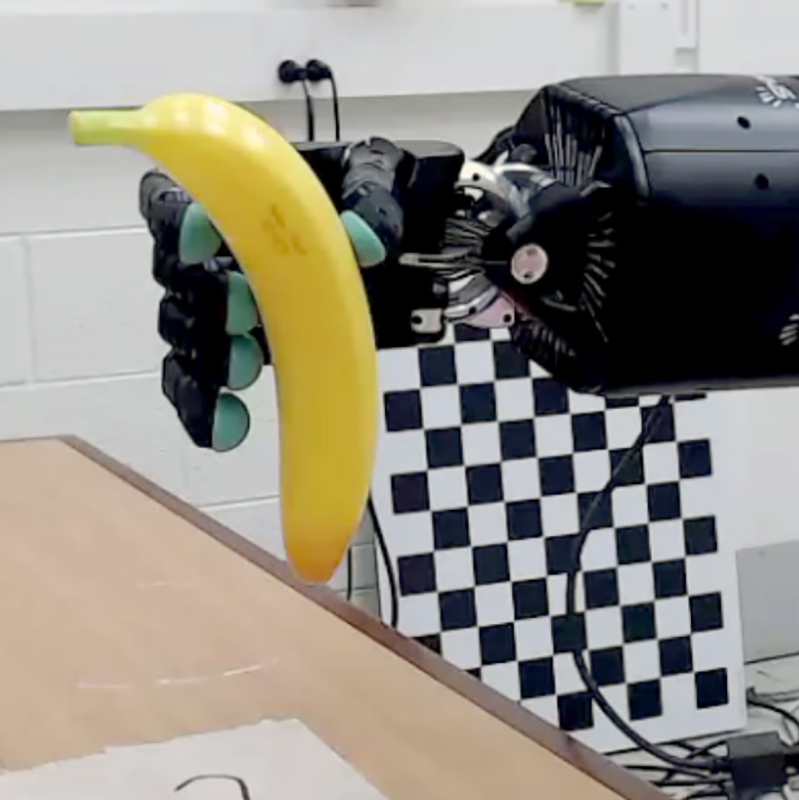
An end-to-end neural network is trained to predict grasp poses for novel objects with which it is unfamiliar. The neural network prediction is adjusted using a differentiable grasp quality metric.
Robots are being used in object packing and dexterous manipulation, and one of the most important challenges they face is grasping unknown objects. In Deep Differentiable Grasp Planner for High-DOF Grippers, ISR-affiliated Professor Dinesh Manocha (ECE/CS/UMIACS), Min Liu, Zherong Pan, Kai Xu, and Kanishka Ganguly present an end-to-end algorithm for training deep neural networks to grasp novel objects.
While robots have been able to generate grasp poses for an arbitrary gripper or target object, they do not do well in the uncertainties of the real world. Recently, learning-based methods have led to improved robustness in handling sensor noise. These methods propose that the robot learns intermediary information such as grasp quality measures and reconstructed 3D object shapes instead of directly inferring the grasp poses. The robot then can use this intermediary information to help it infer grasp poses.
Learning this kind of information can improve both the data-efficacy of training and the success rate of predicted grasp poses. However, intermediary information complicates training procedures, hyper-parameter search, and data preparation.
Ideally, a learning-based grasp planner should infer grasp poses directly from raw sensor inputs such as RGB-D images, and such approaches have been developed by many researchers. However, recent methods show that it is preferable to first learn a grasp quality metric function and then optimize the metric at runtime for an unknown target object using sampling-based optimization algorithms, such as multi-armed bandits.
This optimization can be very efficient for low-DOF parallel jaw grippers but less efficient for high-DOF anthropomorphic grippers due to their high-dimensional configuration spaces. In addition, because it is possible for the sampling algorithm to generate samples at any point in the configuration space, the learned metric function has to return accurate values for all these samples. To achieve high accuracy, a large amount of training data is needed.
Various techniques have been proposed to improve the robustness and efficiency of grasp planners training. For example, data-efficiency of training can be improved by having the neural network recover 3D volumetric representation of the target object from 2D observations. A 2D-to-3D reconstruction sub-task allows the model to learn intrinsic features about the object. However, this method incurs higher computational and memory cost. In addition, compared with surface meshes, volumetric representations based on signed distance fields cannot resolve delicate, thin features of complex objects. Higher robustness can also be achieved using adversarial training, which in turn introduces additional sub-tasks of training and requires new data.
Dinesh Manocha and his colleagues have developed an algorithm that builds all the essential components of a grasping system using a forward-backward automatic differentiation approach, including the forward kinematics of the gripper, the collision between the gripper and the target object, and the metric of grasp poses.
In particular, the authors show that a generalized Q1 grasp metric is defined and differentiable for inexact grasps generated by a neural network, and the derivatives of our generalized Q1 metric can be computed from a sensitivity analysis of the induced optimization problem. They show that the derivatives of the (self-)collision terms can be efficiently computed from a watertight triangle mesh of low-quality. Put together, their algorithm allows the computation of grasp poses for high-DOF grippers in unsupervised mode with no ground truth data or improves the results in supervised mode using a small dataset.
This new learning algorithm significantly simplifies the data preparation for learning-based grasping systems and leads to higher qualities of learned grasps on common 3D shape datasets, achieving a 22% higher success rate on physical hardware and a 0.12 higher value of the Q1 grasp quality metric.
Published February 13, 2020