News Story
Who's walking deceptively? Manocha's team thinks they know.
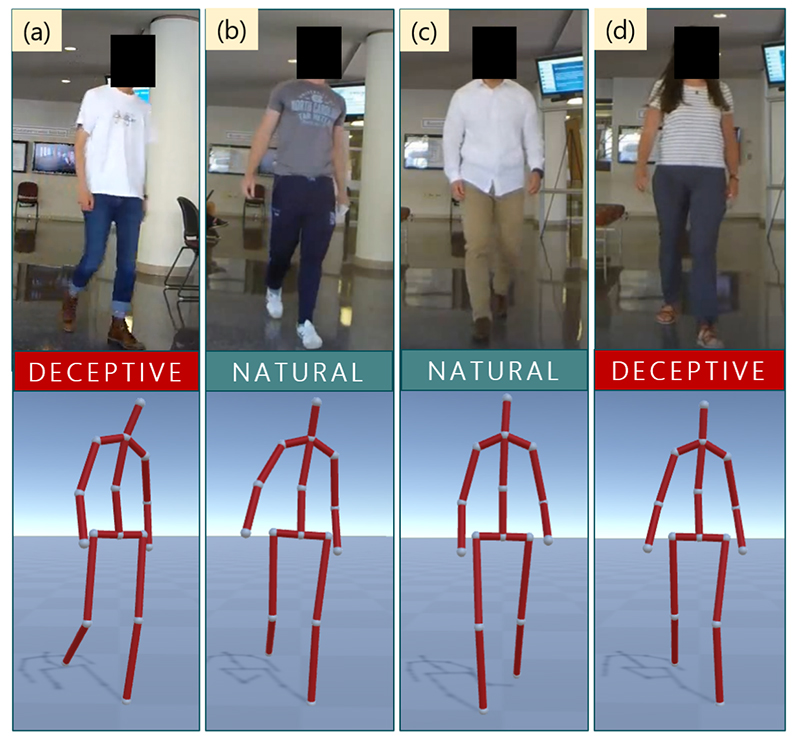
Who’s being deceptive? In (a) smaller hand movements and in (d) the velocity of hand and foot joints provide deception cues.
The Geometric Algorithms for Modeling, Motion and Animation (GAMMA) research team at the University of Maryland and the University of North Carolina has developed a data-driven deep neural algorithm for detecting deceptive walking behavior using nonverbal cues like gaits and gestures.
“The Liar’s Walk: Detecting Deception with Gait and Gesture” is part of GAMMA’s project in social perception of pedestrians and virtual agents using movement features, which it calls GAIT. The researchers include Kurt Gray, Tanmay Randhavane, Kyra Kapsaskis of the University of North Carolina, and Uttaran Bhattacharya, Aniket Bera, and ISR-affiliated Professor Dinesh Manocha (CS/ECE/UMIACS) of the University of Maryland.
In recent years, AI and vision communities have focused on learning human behaviors, and human-centric video analysis has rapidly developed. While conventional analysis of video pays attention to the actions recorded, human-centric video analysis focuses on the behaviors, emotions, personalities, actions, anomalies, dispositions and intentions of the humans in the video.
Surprisingly, detecting deliberate deception has not been a major research focus. Detection is challenging because deception is subtle, and because deceivers are, of course, attempting to conceal their actual cues and expressions. However, much is known about the verbal and nonverbal deception cues of deception. Implicit cues include facial and body expressions, eye contact, and hand movements. Deceivers try to alter or control the cues they believe get the most attention, like facial expressions. Body movements such as walking gaits are less likely to be controlled, making gait an excellent way to detect deceptive behaviors.
The authors developed a data-driven approach for detecting deceptive walking, based on gaits and gestures extracted from videos. Gaits are extracted from videos as a series of 3D poses using state-of-the-art human pose estimation. Various gestures were then annotated. Using this data, the researchers computed psychology-based gait features, gesture features, and deep features (deceptive features) learned using an LSTM-based neural network. The features were fed into fully connected layers of the neural network to classify normal and deceptive behaviors. The researchers trained this neural network “Deception Classifier” to learn the deep features as well as classify the data into behavior labels on a novel public-domain dataset called “DeceptiveWalk,” which contains annotated gaits and gestures with both deceptive and natural walks.
The Deception Classifier can achieve an accuracy of 93.4% when classifying deceptive walking, an improvement of 5.9% and 10.1% over classifiers based on the state-of-the-art emotion and action classification algorithms, respectively.
Additionally, the DeceptiveWalk dataset provided interesting observations about participants’ behavior in deceptive and natural conditions. For example, deceivers put their hands in their pockets and looked around more than participants in the natural condition. Deceivers also display the opposite of an expected behavior or make a controlled movement.
The researchers caution that their work is preliminary and limited in nature, with videos produced in a controlled laboratory setting, and thus may not be directly applicable for detecting many kinds of deceptive walking behaviors in the real world. In addition, while they present an algorithm that detects deception using walking, they do not claim that performing certain gestures and walking in a certain way conveys deception in all cases.
Published January 9, 2020